Paper in press: Barium in seawater ‘solved’
We’re pleased to announce publication of a study led by Öykü Mete (SSF, 2021), published in Earth Systems Science Data. In this study we constrain the distribution of barium in the global ocean using machine learning. We developed thousands of machine learning models that could predict barium concentrations in seawater using GEOTRACES results from in the Arctic, Atlantic, Pacific, and Southern Oceans as training data. We then tested these models by evaluating their ability to predict barium concentrations in the Indian Ocean, noting that no model had seen any Indian Ocean data during training. While we found that many, many models could accurately predict barium distributions in seawater, we focus our attentions on just one, affectionately dubbed model #3080. This model achieves a mean absolute error on dissolved barium concentrations of just 4.3 nmol/kg, equivalent to 6.0 %.
We’re excited about the study for a number of reasons. First, the model provides a global estimate of the total barium inventory of seawater. We calculate this inventory as 122±7 Tmol, which is as much as 21 % lower than what was previously thought. This, in turn, suggests that the residence time of barium in seawater might also be quite a bit lower than current estimates.
Second, we used the model output to explore the distributions of other barium-adjacent properties in seawater, specifically barium-star and the saturation state of seawater with respect to barite. Barium-star illustrates the difference between the observed concentration of barium and that predicted by the concentration of silicon. Saturation state is a measure of whether barite will be thermodynamically inclined to dissolve (values <1) or precipitate (>1) from ambient seawater. Patterns of barium-star and barite saturation state show striking meridional, zonal, and vertical variations that reflect the interplay between ocean physics and biogeochemistry. We then perform some basin averaging and find that the ocean below 1,000 m, is, on a global basis, at thermodynamic equilibrium with respect to barite.
Third, our model illuminates several aspects of the global barium distribution that would have taken years to resolve using direct observations. The predictions can be used to understand barium partitioning into various sedimentary archives, or could serve as ‘targets’ for future process studies. Our research shows that machine learning can make accurate predictions of the chemistry of seawater; moreover, the framework can be used to explore the distribution of other bioactive elements. Watch this space!
If you’re interested in learning more, check out the paper or feel free to explore the data product. You can also download our machine learning model if you want to make your own predictions of dissolved barium concentrations in seawater.
Paper citation: Mete, Ö. Z., Subhas, A. V., Kim, H. H., Dunlea, A. G., Whitmore, L. M., Shiller, A. M., Gilbert, M., Leavitt, W. D., and Horner, T. J. (2023). Barium in seawater: dissolved distribution, relationship to silicon, and barite saturation state determined using machine learning, Earth Syst. Sci. Data, 15(9), 4023–4045, doi:10.5194/essd-15-4023-2023.
Data product citation: Horner, T. J., Mete, Ö. Z. (2023) A spatially and vertically resolved global grid of dissolved barium concentrations in seawater determined using Gaussian Process Regression machine learning. Biological and Chemical Oceanography Data Management Office. Version 2 (2023-07-11), doi:10.26008/1912/bco-dmo.885506.2.
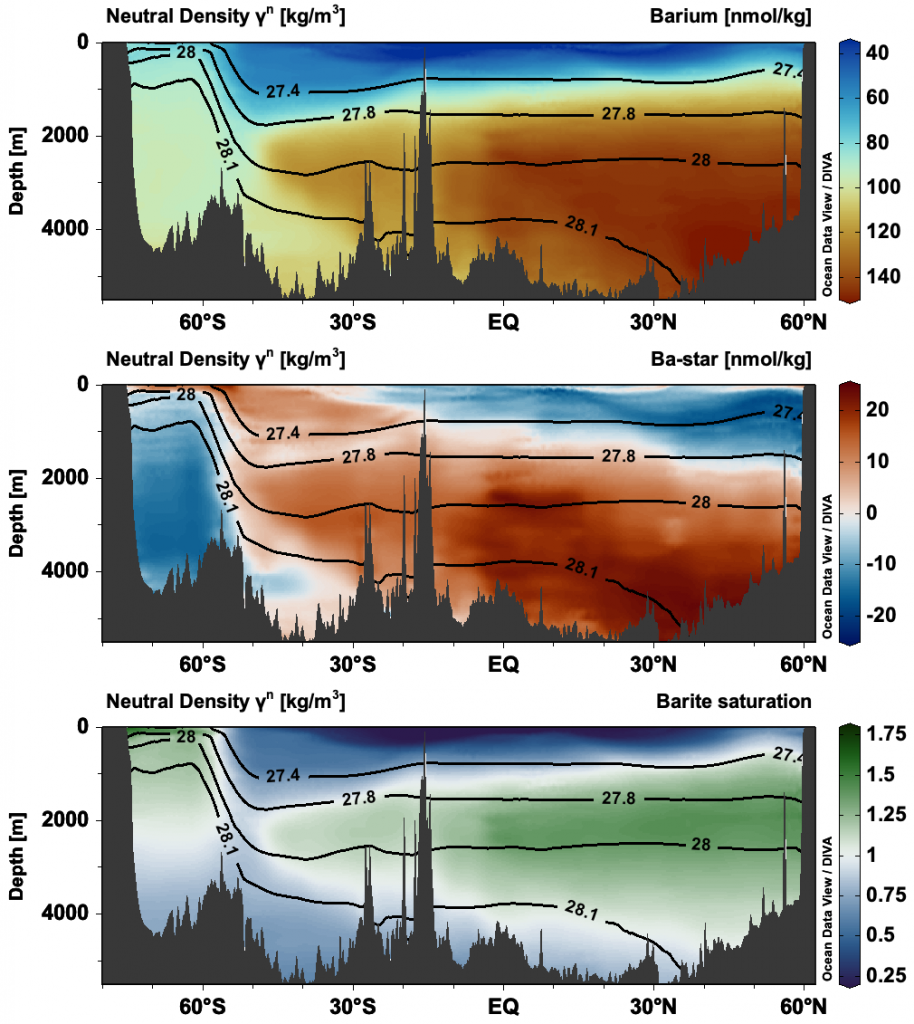
Sections of dissolved [Ba] (top), Ba* (middle), and barite saturation state (bottom) along 145 °W in the Pacific Ocean. Solid lines show neutral density contours.